- Mathematical statistics, inverse problems
-
Estimation and non-parametric tests
- Process and dependency – Extremes – Actuarial statistics
- Bayesian statistics – Learning
Équipe STA
The STA team is part of the Groupe Scientifique Mathématiques de l’Aléatoire (ALEA).
Several research themes are developed within the team. Certain themes attract a majority of members. Others are more marginally studied, but are destined to develop. There are links between all the themes. Such as the use of Bayesian statistics in learning, the construction of non-parametric tests for dependent processes or actuarial models.
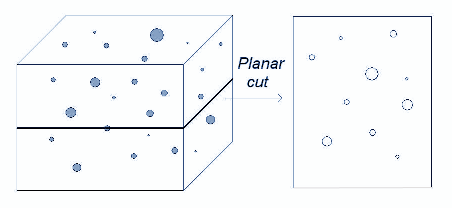
Thèmes de recherche
-
This theme has historically been developed by the Château-Gombert statistics team. Several lines of research are addressed.
→ Aggregation of linear methods using exponential weighting. Although exponential weighting is one of the most effective convex aggregation methods, its rigorous analysis is a generally difficult mathematical problem. A new approach to exponential weighting analysis is developed, based on entropy inequalities for ordered processes. This method would control the concentration of exponential weighting risk in the vicinity of oracle risk ([8] and [19]).
→ Automatic selection of regularization parameters in linear inverse problems. The problem of automatic selection of regularization parameters in inverse problems is one of the fundamental problems in numerical methods. Despite a long history of this problem, there are currently no simple, effective solutions. To build good automatic selection methods, an approach based on a probabilistic interpretation of the inverse problem has been proposed and studied. Specifically, it has been shown that the method based on minimizing the unbiased predictive risk will be close to the optimal method for the inverse problem ([19]).
In [28], theoretical results are obtained on a model generalizing nonparametric multivariate density estimation and multivariate density deconvolution.
We establish lower bounds for the minimax risk under very weak conditions (any function dimension, large regularity space, any Lp loss family). In another work (currently being finalized), we are developing an estimation method that verifies an oracle-like inequality and achieves minimax speeds.Researchers: F. Autin, Y. Golubev, T. Le Gouic, O. Lepski, C. Pouet, T. Willer
-
This line of research can be linked to mathematical statistics, but its developments are numerous enough to be listed separately.
→ Universal estimation procedure. Creation of a universal estimation procedure that simultaneously adapts to the broad spectrum of harmful parameters, regardless of their nature (including structural adaptation). On this subject, collaborations with several researchers from different countries have been successfully completed, with the resolution of numerous problems that had long remained open ([18], [27], [20], [26], [16]). In particular, in the article [24] we find definitive results on the existence of adaptive estimators with respect to the scale of anisotropic Nikolskii classes. In addition, 8 open problems related to adaptive estimation are formulated. This work has highlighted the need to develop new probabilistic tools related to uniform majorants (upper functions) for positive random functionals, see [25],[22], [23], [21], [17].
→ Maxiset approach. The study of maxis performance and estimators using wavelet methods has brought to light new functional spaces, in particular spaces grouping functions with structured wavelet coefficients. The minimax and adaptive minimax speeds of these new spaces are studied.
A work in collaboration with C. Chesneau, [9], concerns the performance of a distribution function estimator in a model of dependent and censored data. The method uses distorted wavelets, and is studied from both theoretical (minimax risk) and practical (estimator behavior on simulated and real data) points of view.
In high-dimensional estimation problems, where the aim is to estimate a d-dimensional parameter from n observations (with d >> n) new methods, such as LASSO or DANTZIG SELECTOR, have recently proved their worth. These methods are all based on solving a constrained l1 optimization problem. It’s a question of using the maxiset point of view to understand whether either of the two methods can be considered the best.→ Nonparametric tests. The variable-weight mixture model is interesting for many problems. The parametric case was discussed in article [3]. The non-parametric case has been treated in several papers to study different aspects: minimax test speed, loss for adaptation: [1], [2] and [4]. This model should be studied as an alternative to imputation methods for dealing with missing data problems (e.g. single imputation, multiple imputation). Another approach is to assume that the variable weights given are not exact, but are subject to error (for example, the variable weights are provided by a human expert or an expert system with training data). The theory of random matrices will probably provide tools to study this problem. Indeed, the behavior of the smallest eigenvalue of a given problem matrix is crucial to the quality of the results obtained.
Researchers: F. Autin, C. Pouet, T. Le Gouic, O. Lepski
→ Long memory process. A series of papers focus on the comparison of processes in different dependency frameworks. We note [13] for the matched case and [12] for the weak dependency cases. Today, we’re interested in building comparison tests for processes with strong dependencies, Gaus- sian fields and stable law processes.
→ Time series. Within the framework of the book “Méthodes en séries temporelles et applications avec R” (Boutahar and Royer-Carenzi), new methods have been developed for the analysis of time series, and in particular the identification of its components. For example, the classic augmented Dickey-Fuller unit root test can distinguish a linear deterministic trend from a stochastic trend of order 1, but fails to detect a deterministic trend if it is of degree greater than or equal to two. We propose a strategy to remedy the situation.
→ Multivariate extremes statistics. As part of Imen Kchaou’s thesis (co-tutored with the University of Sfax), recent work has focused on estimating the dependence function for random variables with extreme laws. We continue to explore the theory of extreme values for time series: asymptotic law of maxima, law of crossing a threshold, asymptotic behavior of estimators of the Pickands function in the case of weak and strong dependence. Extensions to the multivariate case are underway, and there are a number of insurance applications that can be linked to the actuarial work below.
→ Statistical risk modeling. We have studied the approximation of ruin probabilities in the univariate and bivariate cases ([14] and [15]). This is a numerical approach, with the statistical aspect left in perspective for the time being. Multivariate extensions are of particular interest in insurance, as few methods today give satisfactory results for approximating probabilities of ruin. Our polynomial approach seems to give better results. We are also interested in Markov field modeling of mortality.
Researchers : M. Boutahar, D. Pommeret, L. Reboul, M. Royer-Carenzi
These two areas of research have strong links with the key theme of Big Data. The Bayesian aspect has been reinforced by the recent recruitment of P. Pudlo.
→ In Bayesian statistics, several axes are studied: Bayesian variable selection ([7]), ABC-type algorithms ([5]), and the Bayesian approach in Big Data ([6]). Numerous biological applications have been developed.
→ In connection with big data, work is in progress on unsupervised classification methods using decision trees, on extensions to nominal data, and on the importance of variables.
→ Medical modeling work on the development of adaptive questionnaire procedures.
→ Development of discrimination techniques using graphical models with application to medical imaging data.
Researchers: B. Ghattas, D. Pommeret, P. Pudlo
● Interdisciplinary collaboration – Applications
We have several collaborative projects underway. In particular :
– Develop collaborations with geographer colleagues at Aix-Marseille University on transport modeling as part of the development of research at Centrale Casablanca.
– Collaborate with geographers at Aix-en-Provence to model population aging using spatial statistics methods (see [11]).
– Modeling phytoplankton evolution using Bayesian methods (variable selection, mixtures) with the Mediterranean Institute of Oceanography (MIO), in conjunction with M. Dugenne’s thesis and the Amidex CHROME project.
– Extend the work carried out as part of the ECOS 2014 partnership with Uruguay on statistical modeling in ecology.
– Develop Statistical-Signal collaborations, following the example of [10], where C. Coiffard, C. Melot and T. Willer studied a multifractal function by calculating the p-exponents at all points, showing that they are non-trivial.